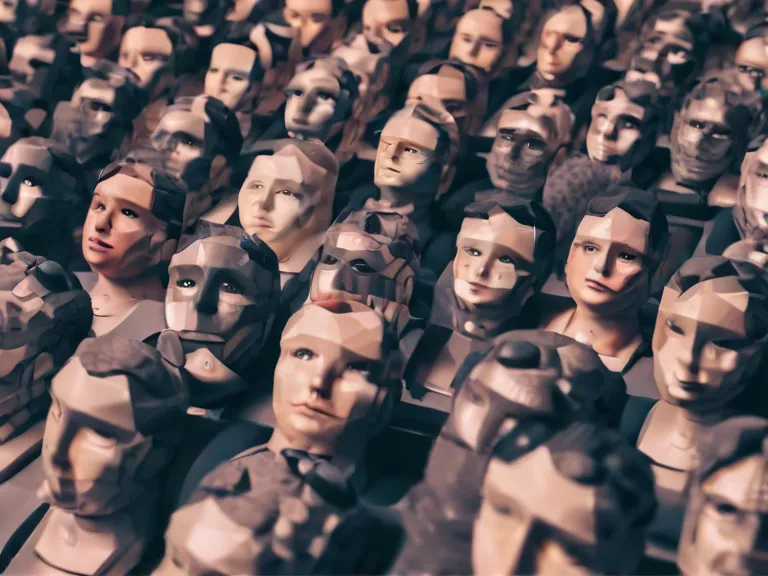
In a world where bias can significantly impact decisions and outcomes, artificial intelligence offers the promise of algorithmic justice. Can AI algorithms ensure fairness in a bias-driven world? Let's explore this question and the potential implications of AI in promoting equality and equity.
AI algorithms have the potential to reduce human bias in decision-making processes by relying on data-driven insights rather than subjective judgments. By analyzing vast amounts of data and identifying patterns, AI can make more consistent and objective decisions. However, this reliance on data also raises concerns about the quality and representativeness of the data used to train these algorithms.
One of the biggest challenges in implementing algorithmic justice is ensuring that AI models are free from biases present in the data they are trained on. Biases in data can perpetuate existing inequalities and discrimination, leading to unfair outcomes. To address this issue, researchers are exploring ways to detect and mitigate biases in AI algorithms through techniques like debiasing and fairness-aware machine learning.
Additionally, transparency and accountability are crucial in ensuring that AI systems promote fairness and ethics. AI developers and organizations must be transparent about the data sources, algorithms, and decision-making processes used in their systems. Moreover, mechanisms for auditing AI systems and holding them accountable for biased decisions are essential for building trust and ensuring algorithmic justice.
While AI offers the potential to enhance fairness and reduce bias in decision-making, it is not a panacea for all societal problems. Ethical considerations, oversight, and ongoing evaluation of AI systems are necessary to prevent unintended consequences and ensure that algorithms promote justice and equality for all.
In conclusion, algorithmic justice through AI has the potential to address biases in decision-making processes and promote fairness in a bias-driven world. However, it requires careful attention to data quality, transparency, accountability, and ongoing evaluation to ensure that AI systems align with ethical principles and societal values.